Pre-print | AfroPALM – Afrocentric Palm oil Adulteration Learning Models: an End-to-end Deep Learning Approach for Detection of Palm Oil Adulteration in West Africa
- Leti Kleyn
- Oct 26, 2024
- 2 min read
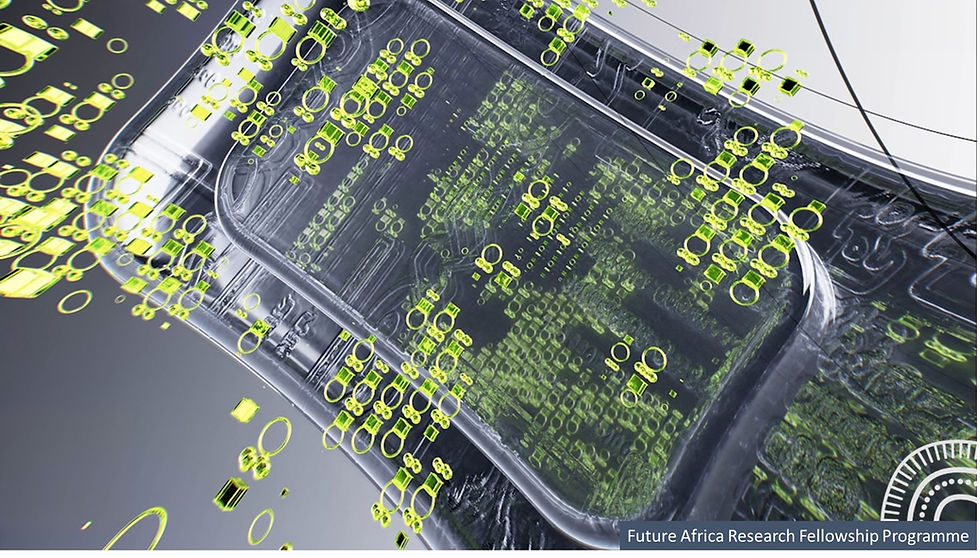
In Africa, rapid population growth and a focus on increasing food production have often overlooked the importance of food safety, leading to the highest per capita incidences of foodborne illness globally. This underscores the imperative to address the food safety challenges on the continent. A critical concern is the widespread adulteration of red palm oil across West Africa, often involving harmful red azo dyes, posing significant health risks. Current detection methods, reliant on laboratory procedures, are time-consuming, expensive and impractical for broad Implementation in local markets. To address these limitations, we propose an end-to-end deep learning approach that bypasses the need for manual feature extraction and laboratory-based analyses. Utilizing high-resolution imaging technology, our approach can objectively and reliably detect palm oil adulteration directly from raw image data. In our study, we developed a deep convolutional neural network dubbed AfroPALM-Custom, specifically designed for detecting palm oil adulteration in African markets. AfroPALM-Custom was pivotal in refining our deep learning approach, achieving a test accuracy of 90.63% and an F1 score of 90.98%. We later adapted mobile-efficient pre-trained models, namely SqueezeNet1. 1 and GhostNetV1—mobile—fine-tuned and dubbed AfroPALM-GhostNet and AfroPALM-SqueezeNet.
Keywords: Food Safety; Food Adulteration; Food Quality; Red Palm Oil; Deep learning
Manuscript submitted to Engineering Applications of Artificial Intelligence | This research received funding from the Future Africa Research Leaders Fellowship (FAR-LeaF) programme, which the Carnegie Corporation of New York funds. This paper was written as part of the Development of an Open Toolbox for Safe Food Monitoring project, which is financed by the FAR-Leaf Programme with technical and Implementation support from the Responsible Artificial Intelligence Lab Project, which is being co-financed by IDRC (Grant Number 109832-001) and GIZ (Agreement Number 81280702)
Preprint publication | Gyabeng, Elton & Tang, Andrews & Agbemenu, Andrew & Odame, Prince & Tchao, E. T. & Keelson, Eliel & Zinia-Zaukuu, John-Lewis & Jerry, Kponyo. (2024). Afropalm – Afrocentric Palm Oil Adulteration Learning Models: An End-to-End Deep Learning Approach for Detection of Palm Oil Adulteration in West Africa. 10.2139/ssrn.4917970: https://rail.knust.edu.gh/2024/10/26/afropalm-afrocentric-palm-oil-adulteration-learning-models-an-end-to-end-deep-learning-approach-for-detection-of-palm-oil-adulteration-in-west-africa/